Matrix operations in R
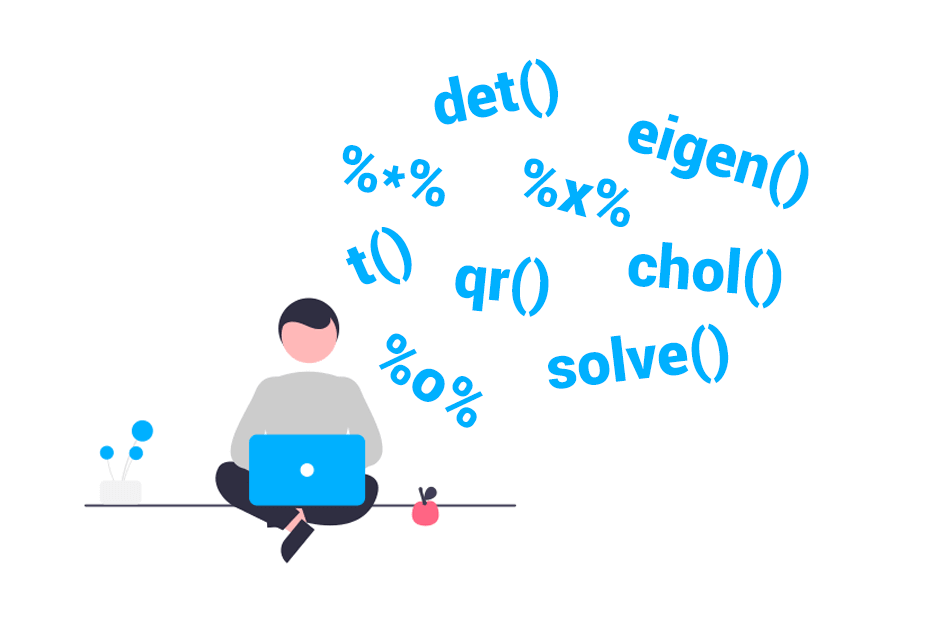
There are multiple matrix operations that you can perform in R. This include: addition, subtraction and multiplication, calculating the power, the rank, the determinant, the diagonal, the eigenvalues and eigenvectors, the transpose and decomposing the matrix by different methods. In this article we will review how to perform these algebra operations in R.
Addition and subtraction
The most basic matrix operations are addition and subtraction. In the following examples we are going to use the square matrices of the following block of code:
A <- matrix(c(10, 8,
5, 12), ncol = 2, byrow = TRUE)
A
B <- matrix(c(5, 3,
15, 6), ncol = 2, byrow = TRUE)
B
# A # B
[, 1] [, 2] [, 1] [, 2]
[1, ] 10 8 [1, ] 5 3
[2, ] 5 12 [2, ] 15 6
These matrices are both of the same dimensions. You can check the dimensions (number of rows and columns, respectively) of a matrix with the dim
function.
dim(A) # 2 2
dim(B) # 2 2
On the one hand, with the +
operator you can compute an element-wise sum of the two matrices:
A + B
[, 1] [, 2]
[1, ] 15 11
[2, ] 20 18
On the other hand, the -
operator will allow you to substract them:
A - B
[, 1] [, 2]
[1, ] 5 5
[2, ] -10 6
Transpose a matrix in R
To find the transpose of a matrix in R you just need to use the t
function as follows:
t(A)
[, 1] [, 2]
[1, ] 10 5
[2, ] 8 12
t(B)
[, 1] [, 2]
[1, ] 5 15
[2, ] 3 6
Matrix multiplication in R
There are different types of matrix multiplications: by a scalar, element-wise multiplication, matricial multiplication, exterior and Kronecker product.
Multiplication by a scalar
In order to multiply or divide a matrix by a scalar you can make use of the *
or /
operators, respectively:
2 * A
[, 1] [, 2]
[1, ] 20 16
[2, ] 10 24
A / 2
[, 1] [, 2]
[1, ] 5.0 4
[2, ] 2.5 6
Element-wise multiplication
The element-wise multiplication of two matrices of the same dimensions can also be computed with the *
operator. The output will be a matrix of the same dimensions of the original matrices.
A * B
[, 1] [, 2]
[1, ] 50 24
[2, ] 75 72
To perform a matricial element-wise multiplication both matrices must be of the same dimensions.
Matrix multiplication
In R, a matricial multiplication can be performed with the %*%
operator.
A %*% B
[, 1] [, 2]
[1, ] 170 78
[2, ] 205 87
Before multiplying two matrices check that the dimensions are compatible. The number of columns of the first matrix must be equal to the number of rows of the second.
Matrix crossproduct
If you need to calculate the matricial product of a matrix and the transpose or other you can type t(A) %*% B
or A %*% t(B)
, being A
and B
the names of the matrices. However, in R it is more efficient and faster using the crossprod
and tcrossprod
functions, respectively.
crossprod(A, B)
[, 1] [, 2]
[1, ] 125 60
[2, ] 220 96
tcrossprod(A, B)
[,1 ] [, 2]
[1, ] 74 198
[2, ] 61 147
Exterior product
Similarly to the matricial multiplication, in R you can compute the exterior product of two matrices with the %o%
operator. This operator is a shortcode for the default outer
function.
A %o% B
# Equivalent to:
outer(A, B, FUN = "*")
, , 1, 1
[, 1] [, 2]
[1, ] 50 40
[2, ] 25 60
, , 2, 1
[, 1] [, 2]
[1, ] 150 120
[2, ] 75 180
, , 1, 2
[, 1] [, 2]
[1, ] 30 24
[2, ] 15 36
, , 2, 2
[, 1] [, 2]
[1, ] 60 48
[2, ] 30 72
Kronecker product
The Kronecker product of two matrices \(A\) and \(B\), denoted by \(A \otimes B\) is the last type of matricial product we are going to review. In R, the calculation can be achieved with the %x%
operator.
A %x% B
[, 1] [, 2] [, 3] [, 4]
[1, ] 50 30 40 24
[2, ] 150 60 120 48
[3, ] 25 15 60 36
[4, ] 75 30 180 72
Power of a matrix in R
There is no a built-in function in base R to calculate the power of a matrix, so we will provide two different alternatives.
On the one hand, you can make use of the %^%
operator of the expm
package as follows:
# install.packages("expm")
library(expm)
A %^% 2
[, 1] [, 2]
[1, ] 140 176
[2, ] 110 184
On the other hand the matrixcalc
package provides the matrix.power
function:
# install.packages("matrixcalc")
library(matrixcalc)
matrix.power(A, 2)
[, 1] [, 2]
[1, ] 140 176
[2, ] 110 184
You can check that the power is correct with the following code:
A %*% A
The matrix must be square to calculate the power, as the number of columns must be equal to the number of rows to compute the calculations.
Note that if you want to calculate the element-wise power you just need to use the ^
operator. In this case the matrix don’t need to be square.
A ^ 2
[, 1] [, 2]
[1, ] 100 64
[2, ] 25 144
Determinant of a matrix in R
The determinant of a matrix \(A\), generally denoted by \(|A|\), is a scalar value that encodes some properties of the matrix. In R you can make use of the det
function to calculate it.
det(A) # 80
det(B) # -15
Inverse of a matrix in R
In order to calculate the inverse of a matrix in R you can make use of the solve
function.
M <- solve(A)
M
[, 1] [, 2]
[1, ] 0.1500 -0.100
[2, ] -0.0625 0.125
As a matrix multiplied by its inverse is the identity matrix we can verify that the previous output is correct as follows:
A %*% M
[, 1] [, 2]
[1, ] 1 0
[2, ] 0 1
Moreover, as main use of the solve
function is to solve a system of equations, if you want to calculate the solution to \(A\) %*% \(X = B\) you can type:
solve(A, B)
[, 1] [, 2]
[1, ] -0.7500 -0.1500
[2, ] 1.5625 0.5625
Rank of a matrix in R
The rank of a matrix is maximum number of columns (rows) that are linearly independent. In R there is no base function to calculate the rank of a matrix but we can make use of the qr
function, which in addition to calculating the QR decomposition, returns the rank of the input matrix. An alternative is to use the rankMatrix
function from the Matrix
package.
qr(A)$rank # 2
qr(B)$rank # 2
# Equivalent to:
library(Matrix)
rankMatrix(A)[1] # 2
Matrix diagonal in R
The diag
function allows you to extract or replace the diagonal of a matrix:
# Extract the diagonal
diag(A) # 10 12
diag(B) # 5 6
# Replace the diagonal
# diag(A) <- c(0, 2)
Applying the rev
function to the columns of the matrix you can also extract off the elements of the secondary diagonal matrix in R:
# Extract the secondary diagonals
diag(apply(A, 2, rev)) # 5 8
diag(apply(B, 2, rev)) # 15 3
Diagonal matrix
With the diag
function you can also make a diagonal matrix, passing a vector as input of the function.
diag(c(7, 9, 2))
[, 1] [, 2] [, 3]
[1, ] 7 0 0
[2, ] 0 9 0
[3, ] 0 0 2
Identity matrix in R
In addition to the previous functionalities, the diag
function also allows creating identity matrices, specifying the dimension of the desired matrix.
diag(4)
[, 1] [, 2] [, 3] [, 4]
[1, ] 1 0 0 0
[2, ] 0 1 0 0
[3, ] 0 0 1 0
[4, ] 0 0 0 1
Eigenvalues and eigenvectors in R
Both the eigenvalues and eigenvectors of a matrix can be calculated in R with the eigen
function.
On the one hand, the eigenvalues are stored on the values
element of the returned list. The eigenvalues will be shown in decreasing order:
eigen(A)$values # 17.403124 4.596876
eigen(B)$values # 12.226812 -1.226812
On the other hand, the eigenvectors are stored on the vectors
element:
eigen(A)$vectors
[, 1] [, 2]
[1, ] -0.7339565 -0.8286986
[2, ] -0.6791964 0.5596952
eigen(B)$vectors
[, 1] [, 2]
[1, ] -0.3833985 -0.4340394
[2, ] -0.9235830 0.9008939
Singular, QR and Cholesky decomposition in R
In this final section we are going to discuss how to perform some decompositions related with matrices.
First, the Singular Value Decomposition (SVD) can be calculated with the svd
function.
svd(A)
$d
[1] 17.678275 4.525328
$u
[, 1] [, 2]
[1, ] -0.7010275 -0.7131342
[2, ] -0.7131342 0.7010275
$v
[, 1] [, 2]
[1, ] -0.5982454 -0.8013130
[2, ] -0.8013130 0.5982454
The function will return a list, where the element d
is a vector containing the singular values sorted in decreasing order and u
and v
are matrices containing the left and right singular vectors of the original matrix, respectively.
Second, the qr
function allows you to calculate the QR decomposition. The first element of the output will return a matrix of the same dimension as the original matrix, where the upper triangle matrix contains the \(\bold{R}\) of the decomposition and the lower the \(\bold{Q}\).
qr(A)$qr
[, 1] [, 2]
[1, ] -11.1803399 -12.521981
[2, ] 0.4472136 7.155418
Last, you can compute the Cholesky factorization of a real symmetric positive-definite square matrix with the chol
function.
chol(A)
[, 1] [, 2]
[1, ] 3.162278 2.529822
[2, ] 0.000000 2.366432
The chol
function doesn’t check for symmetry. However, you can make use of the isSymmetric
function to check it.